A useless non-RIP Gaussian matrix
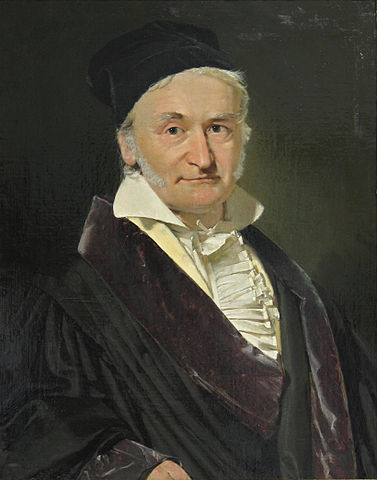
Recently, for some unrelated reasons, I discovered that it is actually very easy to generate a Gaussian matrix \(\Phi\) that does not respect the restricted isometry property (RIP) [1]. I recall that such a matrix is RIP if there exists a (restricted isometry) constant \(0<\delta<1\) such that, for any \(K\)-sparse vector \(w \in \mathbb R^N\), \[(1-\delta)\|w\|^2\leq \|\Phi w\|^2 \leq (1+\delta)\|w\|^2.\]
This is maybe obvious and it probably serves to nothing but here is anyway the argument.
Take a \(K\)-sparse vector \(x\) in \(\mathbb R\) and generate randomly two Gaussian matrices \(U\) and \(V\) in \(\mathbb R^{M\times N}\) with i.i.d. entries drawn from \(\mathcal N(0,1)\). From the vectors \(a=Ux\) and \(b=Vx\), you can form two new vectors \(c\) and \(s\) such that \(c_i = a_i / \sqrt{a_i^2 + b_i^2}\) and \(s_i = b_i / \sqrt{a_i^2 + b_i^2}\).
Then, it is easy to show that matrix \[\Phi = {\rm diag}( c) V - {\rm diag}(s)U\] is actually Gaussian except in the direction of \(x\) (where it vanishes to 0).
This can be seen more clearly in the case where \(x = (1,0,\cdots,0)^\top\). Then the first column of \(\Phi\) is \(0\) and the rest of the matrix is independent of \({\rm diag}( c)\) and \({\rm diag}(s)\). Conditionally to the value of these two diagonal matrices, this part of \(\Phi\) is therefore Gaussian with each \(ij\) entry (\(j\geq 2\)) of variance \(c_i^2 + s_i^2 = 1\). Then, the condition can be removed by expectation rule to lead to the cdf of \(\Phi\), and then to the pdf by differentiation, recovering the Gaussian distribution in the orthogonal space of \(x\).
However, \(\Phi\) cannot be RIP. First, obviously since \(\Phi x = 0\) which shows, by construction, that at least one \(K\)-sparse vector (that is, \(x\)) is in the null space of \(\Phi\). Second, by taking vectors in \(x + \Sigma_K = x + \{u: \|u\|_0 \leq K \}\), we clearly have \(\|\Phi (x + u)\| = \|\Phi u\|\) for any \(u \in \Sigma_K\). Therefore, we can alway take the norm of \(u\) sufficiently small so that \(\|\Phi (x + u)\|\) is far from \(\|x + u\|\).
Of course, for the space of \(K\)-sparse vectors orthogonal to \(x\), the matrix is still RIP. It is easy to follow the argument above for proving that \(\Phi\) is Gaussian in this space and then to use classical RIP proof [2].
All this is also very close from the “Cancel-then-Recover” strategy developed in [3]. The only purpose of this post to prove the (useless) result that combining two Gaussian matrices as in (1) leads to a non-RIP matrix.
References:
- [1] Candes, Emmanuel J., and Terence Tao. “Decoding by linear programming.” Information Theory, IEEE Transactions on 51.12 (2005): 4203-4215.
- [2] Baraniuk, Richard, et al. “A simple proof of the restricted isometry property for random matrices.” Constructive Approximation 28.3 (2008): 253-263.
- [3] Davenport, Mark A., et al. “Signal processing with compressive measurements.” Selected Topics in Signal Processing, IEEE Journal of 4.2 (2010): 445-460.
Image credit: Carl Friedrich Gauss, 1777-1855. Source Wikipedia.