New class of RIP matrices?
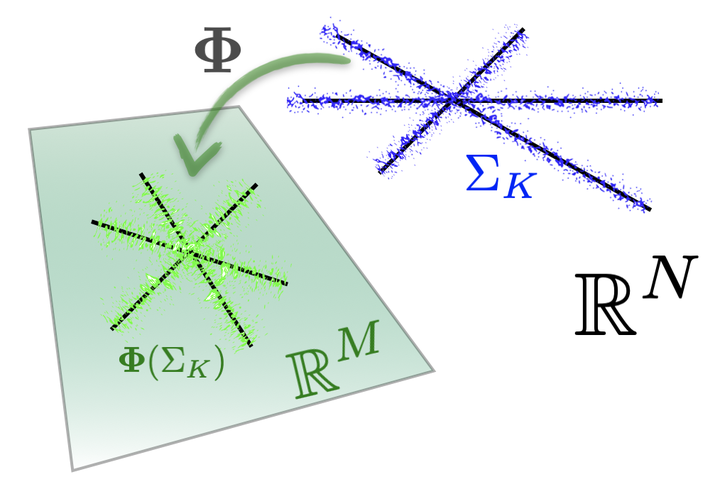
Wow, almost one year and half without any post here… Shame on me! I’ll try to be more productive with shorter posts now ;-)
I just found this interesting paper about concentration properties of submodular function (very common in “Graph Cut” methods for instance) on arxiv:
A note on concentration of submodular functions. (arXiv:1005.2791v1 [cs.DM])
Jan Vondrak, May 18, 2010
We survey a few concentration inequalities for submodular and fractionally subadditive functions of independent random variables, implied by the entropy method for self-bounding functions. The power of these concentration bounds is that they are dimension-free, in particular implying standard deviation \(O(\sqrt{\mathbb E[f]})\) rather than \(O(\sqrt{n})\) which can be obtained for any 1-Lipschitz function of n variables.
In particular, the author shows some interesting concentration results in his Corollary 3.2:
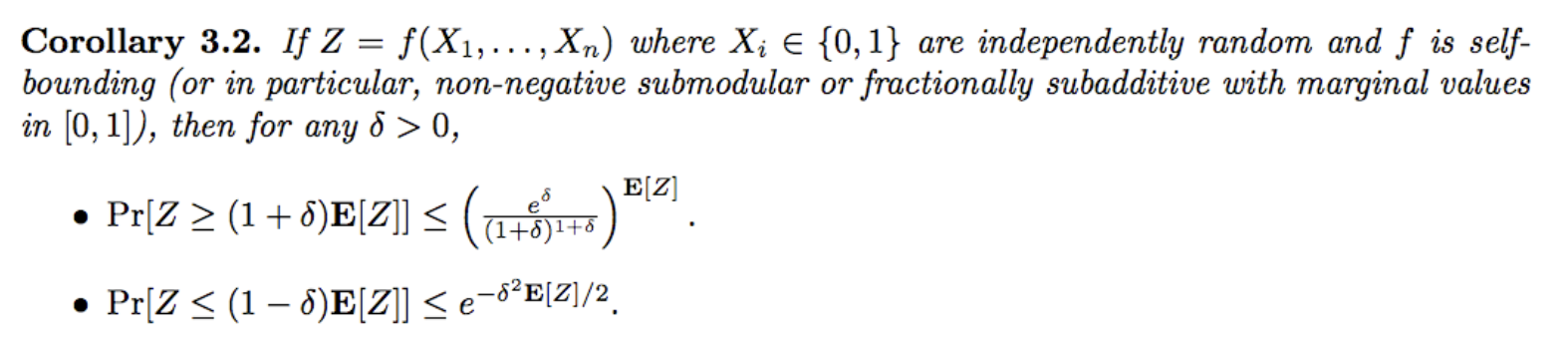
Without having performed any developments, I’m wondering if this result could serve to define a new class of matrices (or non-linear operators) satisfying either the Johnson-Lindenstrauss Lemma or the Restricted Isometry Property.
For instance, by starting from Bernoulli vectors \(X\), i.e., the rows of a sensing matrix, and defining some specific submodular (or self-bounding) functions \(f\) (e.g., \(f(X_1,\cdots, X_n) = g(X^T a)\) for some sparse vector \(a\) and a “kind” function \(g\)), I’m wondering if the concentration results above are better than those coming from the classical concentration inequalities (based on the Lipschitz properties of \(f\) or \(g\). See e.g., the books of Ledoux and Talagrand)?
Ok, all this is perhaps just too hasty thoughts …. written before a mug of black coffee ;-)